Artificial Intelligence within the Rail Industry
Artificial Intelligence (AI) has hit the forefront of business strategy discussions and conference agendas, including the ARDA Annual Meeting in July 2024. The maturity of cloud processing and computing power is enabling AI adoption to grow faster than ever. Companies have access to an unprecedented amount of data, which is fueling the growth of AI technologies. This article will examine how AI is impacting the rail industry now and what to expect into the future.
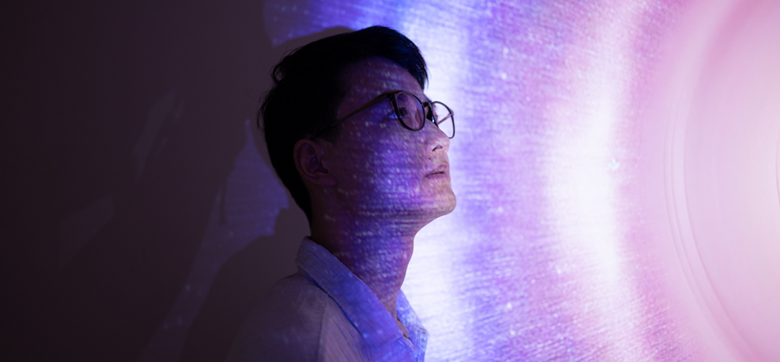
AI Overview
AI can mean different things to different people, largely because AI is somewhat of an umbrella term for a few different types of technologies. Generally, AI refers to anything that allows a machine to do something that ordinarily requires human intelligence, such as recognizing objects, understanding natural language, or solving complex problems. AI technologies mimic human intelligence using math and logic to process data, make predictions or take actions based on data patterns, and learn from its errors to continually improve accuracy. There are distinctions we can make to clarify different subsets of AI technology.
One large-scale distinction is between Traditional AI and Generative AI. The main difference between traditional AI and generative AI is traditional AI systems are primarily used to analyze data and make predictions, while generative AI goes a step further by creating new data like it’s “training data” (i.e., data that it has access to).
- Traditional AI focuses on performing a specific task intelligently. Traditional AI systems have the capability to learn from data and make decisions or predictions based on that data. For example, with a game of chess, a computer can be trained to understand the rules, predict your moves, and make its own moves based on a pre-defined strategy, but cannot develop new strategies or re-invent the game. Real world examples include voice assistants (e.g., Siri or Alexa), recommendation engines (e.g., Netflix or Amazon), or Google’s traditional search engine.
- Generative AI is the next generation of AI, which is a form of AI that can create something new. ChatGPT technology is a great example of this, where you can ask the engine to create a story, draw a new picture, create a song, or summarize documents. Generative AI technology does this by looking at a large number of existing examples, also known as ‘training data’.
Another distinction is by subsets of AI technology like Machine Learning, Language Models, Neural Networks, and Robotics. Each subset can use either traditional AI, generative AI, or a mix of both. These categories have some overlap in applications but can be helpful to think about how different AI processes are applied.
- Machine Learning is a type of AI that enables a machine to learn on its own by analyzing training data, so that it can improve its performance over time. Examples include object tracking, image classification, and facial recognition. For the rail industry, examples include using satellite or drone imagery to look for vegetation crowding tracks (image classification) or autonomous rail car technology (object tracking).
- Language Models are machine learning models with the ability to understand and generate human language. Language models are used in LLM (Large Language Model) and NLP (Natural Language Processing). Language models are trained on large amounts of data, such as books, articles, and web pages to learn patterns and connections between words and phrases so they can generate new content. Examples include virtual chatbots, virtual assistants, and ChatGPT tools. For the rail industry, this includes using ChatGPT tools to analyze and summarize documents, and tools to generate code for different software platforms.
- Neural Networks are a type of machine learning that can make decisions like the human brain by using processes that mimic the way biological neurons work together to solve complicated problems like summarizing documents or recognizing faces with greater accuracy. Examples include computer vision, speech recognition, and more advanced language processing. For the rail industry, examples could include generating financial predictions by processing historical financial data, applications for quality control, or asset management optimization.
- Robotics is a type of AI focused on machines that can assist people with little or no human involvement. For example, robotics could be used for track maintenance or manufacturing.
AI Evolution
AI capabilities have accelerated in recent years due to decreasing costs of data storage and processing, rapidly expanding data availability, and improved data storage and modelling techniques. Many sources cite generative AI building momentum in 2017 and hitting an inflection point at the end of 2022 when applications such as ChatGPT became publicly available. As of 2024, many individuals and companies are exploring AI technology embedded in common software platforms like Microsoft, Amazon, Apple, Google, and IBM.
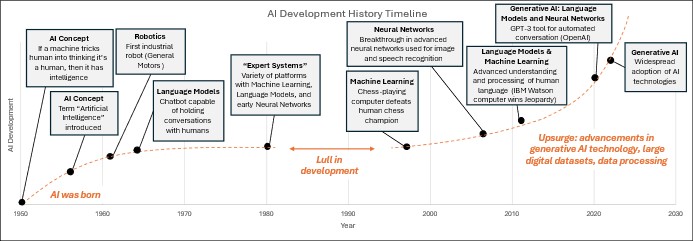
Like many industries, the rail industry has faced challenges in adopting AI technologies due to limited data availability and quality to use for training data. Today, the rail industry is positioned well to adopt more AI technologies due to AI advancements, access to AI tools, improved data practices, and increased digital skills and knowledge from the rail industry workforce.
While there are many ways to define AI, the more important conversation revolves around what AI enables us to accomplish.
AI Use Cases in the Rail Industry
The impact of these technologies stretches beyond just the operating elements of the rail industry and to areas including real estate, environmental concerns, legal matters, and economic growth.
A few examples of use cases were mentioned above. Some others include:
- Improving efficiency of workers
- Predictive maintenance
- Train or track inspections
- Optimization of services, logistics, compliance, safety protocols
- Improving report-generation efficiency
- Data analysis and trend identification
- Performance evaluation
- Financial planning and forecasting
- Prioritization of key business objectives such as performance, safety, and operational efficiency.
Benefits and Risks
While new technologies offer significant benefits and advancements, with any innovation comes inherent risks that must be carefully evaluated and addressed.
Risks associated with AI include the potential for “hallucinations” (incorrect or misleading results) when AI tools lack adequate training data, leading to errors or incorrect outputs that require human intervention to rectify. There can also be a challenge of a shortage of high-quality training data and the inability of models to distinguish between credible and outdated sources, which can augment issues of inaccurate results. Specialty applications for fields within the rail industry (e.g., environmental remediation) could be more prone to these errors since training data are limited. In AI development, risks involve addressing biases, promoting inclusivity, safeguarding privacy, ensuring data security, and maintaining transparency in decision-making processes. Legal concerns can include understanding the ownership of AI-generated outputs, which can be defined by each AI tool’s ownership policies but often can be open to interpretation. Many companies are looking at shifting to secure internal networks to control data security, where AI data sources, processing, and output are all contained in a confidential environment.
Despite these challenges, the benefits of AI adoption include enhanced efficiency, improved accuracy in decision-making, and new solutions or automations.
The Future of AI
AI will continue to advance significantly in the coming years, bringing many applications across different areas of the rail industry. In the short term, the focus will be on efficiency gains in routine work, better access to historic data, and image processing. This includes leveraging and adapting to Machine Learning and Language Model tools in the way we work. The best near-term opportunities for AI are not necessarily outside-the-box or difficult to find. We can find short-term gains by looking critically at tasks that we all know are difficult, time-consuming, and expensive. Longer-term possibilities include generative AI like solution suggestions, tools for financial planning and portfolio management. Embracing AI in the rail industry will not only streamline operations but also generate opportunities for innovation and cost savings by reevaluating challenging and resource-intensive tasks. Some companies have seen good results by supporting voluntary employee learning and engagement with AI, creating opportunities for training and experimentation within safe, controlled environments and developing a sense of involvement and opportunity around new tools. Ultimately, the key lies in aligning AI capabilities with specific business goals to fully leverage its transformative potential.
Summary
AI technology includes powerful tools that are starting to transform the industry as we know it and will touch all of us professionally. There has been a notable surge in the adoption and utilization of AI technologies over the last few years. Despite being in its early stages, companies are actively engaged in the development of AI applications and exploring diverse use cases, underscoring the potential this technology holds.
References
- The six main subsets of AI: (machine learning, NLP, and more), Akkio (January 2024)
- What is artificial intelligence? The difference between generative AI and traditional AI: an easy explanation for anyone, Forbes (August 2023)
- 74 years: a Timeline of Artificial Intelligence Developments, SYNCOG (September 2024)
- The journey toward AI-enabled railway companies, McKinsey & Company (March 2024)
- Artificial intelligence and what it means for the rail industry, RT&S (March 2024)